The High-Uncertainty Challenge of Tech Development
- Colin Swindells
- Dec 18, 2024
- 4 min read
This article shows two use cases to show the challenges of estimating and managing complex tech projects, particularly in contrast to more predictable operational tasks. By understanding the inherent uncertainties in software development, leaders can make more realistic plans, allocate resources effectively, and set realistic expectations. The article delves into the importance of agile methodologies in managing complex projects. #softwareengineering, #productmanagement, #projectmanagement, #engineeringmanagement, #techleadership, #innovation, #agilemethodologies, #workplaceculture, #productivity, #businessstrategy
Annie, a comptroller at a 100-person tech startup, complained “Why are the software engineers always behind?” “Why can’t they better estimate their time?” “Why can’t the product managers do their jobs better?” These are common critiques that I’ve heard at virtually every innovative tech company where I’ve worked. Communicating the relative uncertainties often helps build understanding and empathy for the challenges typically experienced by product innovators, such as engineers, scientists and researchers, thereby setting up the company executives for success.
Figure 1 compares two use cases: one with high uncertainty and one with low uncertainty. This is based on the observation that many innovative tech development tasks have high levels of intrinsic uncertainty that can not be known ahead of time or figuring out a resource estimate would take too many resources – possibly many more resources to estimate than just doing the task. Conversely, many operational tasks have low levels of uncertainty. First, a use case for a typical software engineering activity of updating an AI algorithm is shown. Second, a use case for a typical comptroller activity of tax preparation is shown.

For the sake of easy comparison, suppose the software engineer and comptroller compensations are the same at the company, with mid-level and senior-level rates of $100 / hr and $200 / hr. For the engineer’s task estimate of 1 day, the task could end up taking 10x more or less time than estimated. Unforeseen debugging, troubleshooting, problem complexity, changing requirements, evolving technologies, interdependencies and other challenges all contribute to a high amount of intrinsic uncertainty. Furthermore, a more experienced engineer may have more difficulty estimating time (not less) because such an engineer will typically work on the most challenging, and therefore often most uncertain, tasks. For the comptroller’s estimate of 1 day, the task could end up taking 0.5x more or less time than estimated. Tax preparation is a highly operationalized activity with well defined rules and structure. Tax activities from last year and the years before serve as excellent indicators of the resources needed to do taxes in the current year. Uncertainties include changes to the tax code and error-checking financials submitted by various staff.
For this example of 1 day’s worth of work, we can see that if the comptroller gets very behind, she can still make her deadline. For example, being behind her full 0.5x bound results in her being behind her estimate by 0.5 days (4 hours in a typical 8-hour work day). Worst case, she can spend a few hours in the evening to make up for the uncertainty. Or, the typical suggested traditional management approach of buffering time could work well. Conversely, suppose the engineer discovers an unforeseen dependency after 7 hours of work that takes 10 days to fix. No all-nighter coding marathon can pack 70+ hours of coding into a single evening! And, fully buffering timelines for most engineering uncertainties would not be feasible or accepted by the company’s financial leaders.
Figure 2 extends the uncertainties in Figure 1 for a typical two-week sprint of 10 work days. The engineering uncertainty has a striking range of 1 to 100 days whereas the comptroller uncertainty has a range of 5 to 15 days. To make matters worse, the high uncertainty tasks are often much more interdependent than the low uncertainty tasks. Consequently, adding more engineers tends to make the resourcing problems worse, not better. See the classic Mythical Man Month for details [1]. Conversely, low uncertainty tasks of more operationalized activities, like tax preparation, are more likely to be cleanly chunked into different discrete sub-tasks for parallel processing by temp staff support.
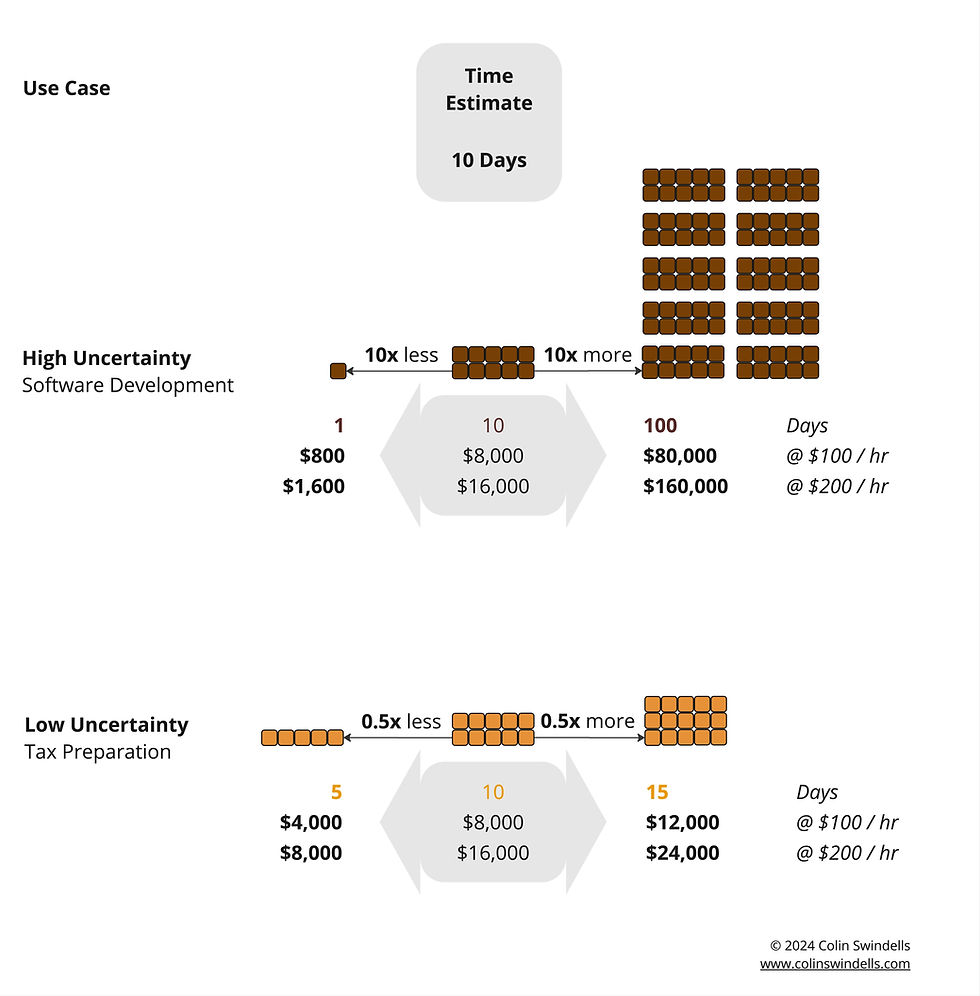
The above two use cases visually show why more agile work processes are more effective for what Eric Schmidt, Jonathan Rosenberg, and Alan Eagle from Google call creative mode activities [2], such as innovative engineering, compared to operational efficiency activities, such as scaled engineering or tax preparation. See Will Larson’s An Elegant Puzzle [3] for one the best detailed descriptions of the challenges and demands of engineering management to address the high uncertainty situations shown in Figures 1 and 2.
[1] Brooks FP. The mythical man-month. Datamation. 1974 Dec;20(12):44-52.
[2] Schmidt E, Rosenberg J, Eagle A. Trillion Dollar Coach. The Leadership Playbook of Silicon Valley’s Bill Campbell. HarperCollins; 2019 April 16.
[3] Larson W. An Elegant Puzzle: Systems of Engineering Management. Stripe Press; 2019 May 20.
Comments